FinTech is transforming industries, boosting productivity, and redefining business operations in today’s data-driven world. At Unleashing Innovation: FinTech Forward, global leaders and financial professionals will explore cutting-edge breakthroughs, explore market opportunities, and tackle Canada’s productivity challenges.
Among the lineup of world-class speakers is Dr. Marcos López de Prado, a trailblazer in quantitative finance and financial data science. Here’s our conversation with Dr. López de Prado ahead of the event, where he shares how AI is reshaping finance, why human roles are evolving from decision-making to system design, and what the future holds for a more inclusive and efficient financial ecosystem.
Artificial intelligence has become a buzzword in many industries. From your perspective, what makes finance such fertile ground for AI innovation?
Finance involves making decisions under uncertainty. This uncertainty is due to numerous factors, such as making predictions about the true state of the economy, potential future outcomes, relative valuations, etc. To mitigate this uncertainty, economic agents gather and process large amounts of information. Some 50 years ago, when information was still relatively limited and it disseminated slowly, humans could make informed decisions and act fast enough before prices reflected that information. In contrast, today information is plentiful, and it disseminates quickly. As a result, human decision makers are at a disadvantage to algorithmic decision makers. This does not mean that there is no role for humans, but that the role of humans is quickly evolving, from decision-makers to system designers, operators and overseers.
Your groundbreaking work has applied machine learning to solve some of the most complex problems in institutional investing. And, you’ve emphasized the importance of AI in reducing inefficiencies in financial markets. Can you share an example of how this has been achieved in practice?
When I joined the investment industry, some 25 years ago, trading was a painful process, plagued with excessive costs, inefficiencies, delays, abuses, and errors. Retail investors were at the mercy of brokers. That began to change around 2005, with the onset of high-frequency trading (HFT). HFT is the result of three innovations:
- democratization of market access, which resulted in increased competition among market participants;
- technological progress in exchange connectivity, which allowed prices to reflect information faster; and
- advances in market microstructure theories and AI that allowed market participants to model prices and risks better (such as the VPIN theory, which I developed with professors Maureen O’Hara and David Easley to help market makers provide liquidity more efficiently).
As a result, today’s retail investors can buy securities at a fraction of the prior-HFT cost, with the confidence that most inefficiencies have been arbitraged away. This is not to say that HFT is perfect, but most experts agree that it has benefited investors as a whole.
Many financial institutions struggle with the “curse of dimensionality” when applying machine learning. Can you talk a bit about what this is, and what strategies do you recommend for handling large and complex datasets effectively?
The “curse of dimensionality” refers to the challenges that arise when the number of dimensions in a dataset grows large, often exponentially increasing the complexity of machine learning models. This phenomenon can lead to overfitting, poor generalization, and computational inefficiency, as models struggle to extract meaningful patterns from sparse and noisy data in high-dimensional spaces.
There are some strategies to address the curse of dimensionality and handle large, complex datasets effectively:
- First, dimensionality reduction techniques allow users to select the most relevant features in the data and transform datasets to lower-dimensional spaces.
- Second, regularization techniques introduce penalties on complexity to the model to reduce overfitting.
- Third, cross-validation and out-of-sample testing apply techniques that estimate how well the model performs on new data unseen by the model.
- Fourth, causal discovery analysis untangles the web of dependencies in the data, which can be used by researchers to inform their model choices.
By applying these strategies, institutions can mitigate the challenges posed by high-dimensional datasets, allowing machine learning models to extract valuable insights and make robust predictions.
What specific breakthroughs in machine learning do you believe have the greatest potential to revolutionize fintech and financial services in the near future?
As machine learning helps increase the productivity of our labor force, it will become more important that these systems are trustworthy at different levels, including robustness, explainability, and accountability. A system is robust when its decisions are reproducible, not overly sensitive to small changes in its inputs, and incorporates safeguards against misuse. Explainability means that the system’s decisions are transparent and understandable to users and stakeholders. There is accountability when the system’s errors can be assessed and corrected, and there are protocols for assigning responsibilities.
In your view, how can organizations leverage machine learning to gain a competitive edge?
Organizations must retrain their workforce so they can learn to capture, process, structure, analyze, and model data. It is not necessary for all workers to become programmers, but they should know enough to interact effectively with the algorithms they will need to be more efficient in their roles. This is the “centaur paradigm” of a human-algorithm hybrid, which I described in my testimony before the United States Congress in December 2019.
Consider a radiologist working in a hospital. Various algorithms are capable of detecting the presence of cancer in an X-ray with greater accuracy than a team of radiologists with decades of experience. These expert radiologists should familiarize themselves with how these algorithms operate, understand what they do better than humans, why they sometimes make mistakes, and learn how to complement them. The best radiologist of the future will be the one who knows how to use these algorithms most effectively. The same can be said of most professions.
Looking ahead, what role do you see AI playing in solving systemic challenges, such as improving financial inclusion or addressing market volatility?
AI has tremendous potential to address these challenges. For financial inclusion, AI can use non-traditional data to assess creditworthiness, enabling access to financial services for underserved populations. It also lowers operational costs, making microfinance and tailored solutions more accessible, even in remote areas.
In tackling market volatility, AI excels at identifying early warning signs through predictive analytics and managing risk with real-time stress testing and algorithmic trading. It can stabilize markets by mitigating inefficiencies and ensuring liquidity. An example of this is the VPIN theory, as I mentioned earlier.
However, the key is transparency and ethical deployment. Trust in AI systems, coupled with robust governance and education to empower workers, will be critical. Ultimately, AI is a tool to augment human expertise, not replace it, and its success lies in thoughtful integration into financial ecosystems.
For attendees of our upcoming event who are looking to stay ahead in this rapidly changing landscape, what key skills or knowledge areas should they prioritize?
To stay competitive, attendees should focus on data literacy—understanding how to capture, process, and analyze data effectively. Familiarity with AI and machine learning concepts is also crucial, even if they’re not programmers, as it helps them work seamlessly with advanced tools.
Additionally, they should develop skills in critical thinking and decision-making, learning how to complement algorithms with human judgment. Understanding ethical AI practices and regulatory compliance will also be essential as technology evolves. Ultimately, the ability to adapt, learn, and collaborate with both humans and algorithms will define success in this dynamic environment.
Dr. López de Prado is a pioneer in the fields of quantitative finance and financial data science. A professor of practice at Cornell University and Global Head of Quantitative Research and Development at the Abu Dhabi Investment Authority (ADIA), his work has reshaped how financial markets are analyzed and investment funds are managed. While his algorithms have helped automate many financial tasks, he has also been a strong advocate for AI that is transparent, ethical and accountable to humans.
Marcos’s contributions have earned him prestigious accolades, including his recent appointment as Knight Officer of the Royal Order of Civil Merit by King Felipe VI and the Government of Spain, “for distinguished services to science and the global investment industry.”
Whether you’re a seasoned professional or a newcomer to the fintech space, don’t miss the chance to engage with thought leaders, explore actionable strategies, and stay ahead in this dynamic industry. Join the conversation, and together, let’s drive the next wave of financial innovation.
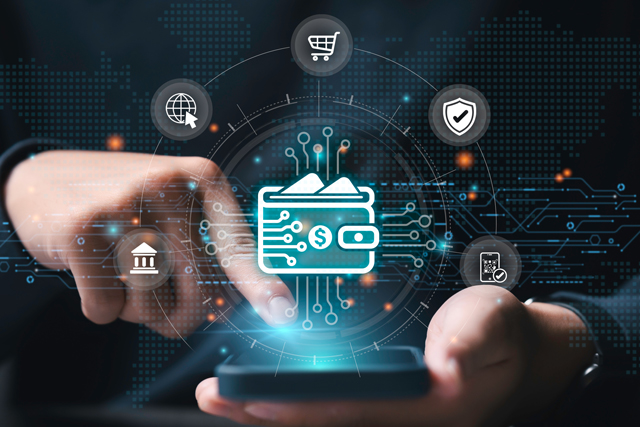
Unleashing Innovation: FinTech Forward
8 AM - 3:45 PM